Structural biology in the era of artificial intelligence: Excitement and pitfalls
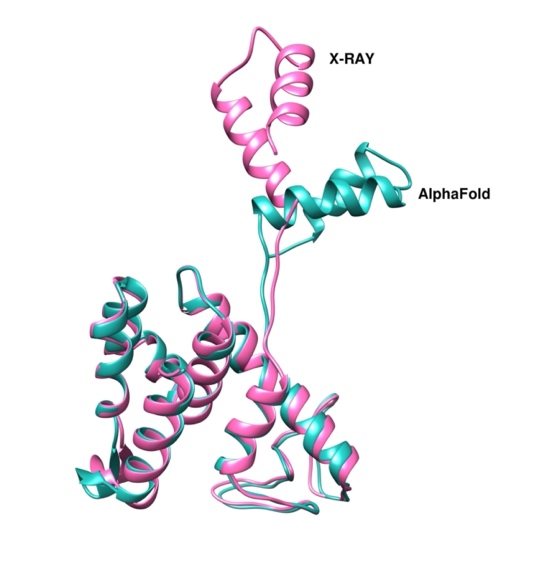
The accurate prediction of the shape (structure) of a protein from just its amino acid sequence, the so-called ‘protein folding problem’, has been one of the ‘Holy Grails’ of science and a computational challenge for the last 50 years or so. Recent advances, however, in the field of artificial intelligence have led to a breakthrough in protein structure predictions, creating a lot of excitement amongst researchers but also raising questions about the future of structural biology, in particular the need for experimental structure determinations.
The interest in protein structures stems from the fact that knowing the shape of the proteins is essential in understanding life. Proteins are important molecules for all living organisms and responsible for almost every biological process. They can function in specific ways and, according to an old axiom of molecular biology, the function of each protein is determined by its 3-dimensional structure. Thus, the shape of a protein will determine if that protein is, for example, an enzyme with a particular specificity, a transport protein, or a scaffold protein. Following laws of physics and chemistry, and sometimes with the help of other proteins called chaperones, proteins take their final shape inside the cells where they are initially synthesized. If proteins do not fold up properly, they can accumulate inside the cells, resulting in neurogenerative diseases and ageing.
The challenge to solve the ‘protein folding problem’ has been best illustrated by the Levinthal paradox. Assuming a protein with 100 amino acids, the number of conformations is so large (Cyrus Levinthal calculated some 10300 conformations in one of his papers) that in order for a protein to go through all the available conformations ‘till it finds the correct one it would require more time than the age of the universe (13.2 x 109 years). In cells, however, proteins are able to find easily and fast their correct conformation and fold very efficiently in milliseconds and sometimes in microseconds.
Various computational approaches have been used over the years to solve the ‘protein folding problem’ and predict protein structures solely from the amino acid sequence. To assess the progress of the computational programs in making the right predictions, a biennial competition known as Critical Assessment of Structure Prediction (CASP) was established in 1994 to evaluate progress in the accuracy of computational protein structure predictions. During the CASP competitions, target proteins are released over a period of several months and participating teams have several weeks to submit their predictions. A team of independent scientists then assess the quality of the predictions comparing them with the actual experimental structures. The CASP competition uses the global distance test (GDT) metric to assess accuracy. GDT measures how closely the predicted shape of a protein matches the shape from experimental methods. Any program reaching a score of around 90 GDT is considered to be competitive with experimental methods. As announced at the beginning of December 2020, in the latest CASP meeting (CASP14), an artificial-intelligence company, DeepMind, was able to train its deep-learning algorithm called AlphaFold2 to predict protein structures with a precision comparable to that of experimental methods. AlphaFold2 achieved a median score of 92.4 GDT across all targets, leaving all its competitors far behind. The software was trained on around 170,000 structures that were present in the Protein Data Bank (PDB). The accuracy, therefore, is comparable to experimentally determined structures, for example using X-ray crystallography and cryo-electron microscopy (cryo-EM).
Artificial intelligence has made great steps in recent years. It is estimated that in 2020 there were about 21,000 papers related to AI. This number is expected to grow by 50% every year. Most work on AI is being done by tech companies, which do not publish their code. Only 25% of AI papers publish their code. Thus, a major concern has been if DeepMind will decide to release the code and make it freely available or opt out to commercialize on the findings. Google, which owns DeepMind, has made a big investment in AI with some heavy losses amounting to ~1.5 billion dollars in 2019. However, in a big step recently, DeepMind teamed up with the European Bioinformatics Institute to create a database (AlphaFold DB; https://alphafold.ebi.ac.uk) of predicted models from the human proteome and the proteome of 20 other organisms of significant medical or biological interest. Moreover, the company plans to expand the AlphaFold DB in the coming months with inclusion of millions more predicted models (currently 992,316 predicted structures as of March 30th, 2022). It should be noted that the PDB contains over 180,000 entries which cover ~55,000 unique proteins (UniProt accessions). This number is almost a thousand times lower that the current sequences found in UniProt (~220 million sequences). Thus, the limited coverage in the PDB of the protein universe is currently an impediment for many areas of biology, including for structural biology itself. In addition, predictions can now be easily done by everyone using a web browser by simply giving the sequence of the protein of interest.
The availability of a large number of predicted models can help the structural biology community in various ways. The ability to predict protein structures will, for example, accelerate the evolutionary analysis of proteins using the available genomic data to shed light on the function of thousands of proteins in the human genome whose structure are currently unsolved. The effect of gene variations in diseases among people would be also easily studied without the need to obtain experimental structures. The predicted models can be used, for example in structure determination efforts to provide phases in molecular replacement. Use of predicted models could be particularly beneficial in determining structures for which efforts to solve them have failed in the past. In crystallography or cryo-EM, poor defined regions owing to increased flexibility could be more easily built with the help of a predicted structure. Models can be used also to identify functional domains in proteins to assist in cases where expression of the full-length protein is problematic. In this way, functional domains could be expressed separately or in combination with other domains.
There are areas, however, that call for some skepticism. The targets at CASP competitions are single proteins or domains and not protein complexes. The algorithm has been not very efficient in modeling individual structures in protein complexes, or groups, whereby interactions with other proteins distort their shapes. This is a field considered as the next frontier for DeepMind where improvements to understand better how proteins form complexes or how they interact with small molecules are needed.
Proteins are like living organisms. They are not a static or rigid assembly of amino acids in a 3D-space. They can change shape, take different conformations depending on their environment or the work they need to perform, and adjust their shape according to substrates and ligands they have to bind. In these cases, a better understanding of how proteins work requires molecular dynamics studies and various experimental structures under different conditions to characterize all different states a protein can take (Fig. 1). Structural predictions could therefore be a good starting point to understand a protein and to get the first structural snapshot but, most likely, not the ‘end of the story’.
The binding of ligands or drugs to a protein would probably require better precision that the current one. The average or root-mean-squared difference (RMSD) in atomic positions between the prediction and the actual structure is 1.6 Å (0.16 nm), which is roughly the size of a bond-length. This number refers only to the backbone atoms of the protein. While that is good enough for producing an accurate picture of the overall fold of the protein, it doesn’t provide information about how well the positions of the side-chain atoms are predicted. Therefore, the average difference in atomic positions for all atoms (backbone and side-chain) is likely to be much greater than 1.6 Å. A drug discovery effort requires a confidence in atomic positions within a margin of <0.3Å, which currently cannot be delivered byAlphaFold2 predictions.
Another problem arises from the basic idea of AI. In order to learn better the rules of protein folding, an AI network requires continuous training on existing protein structures. Thus, it may be more difficult for AI to predict the structures of proteins with folds that are not well represented in the PDB. Besides, if experimental structures are no longer determined and all structural information is obtained through AI, this would create problems in training the AI network and trusting the accuracy of the predictions. After all, we all learn from experiments, and this is no exception even for AI.
Despite the limitations, structural predictions could have a big impact on protein research. There are many things to learn regarding proteins and how they work and communicate. Further development will certainly improve the accuracy of predictions. In the meantime, the predicted models can certainly help in designing new experiments for better characterization of proteins, assisting in experimental structural biology techniques, such as X-ray crystallography and cryo-EM, to solve new and difficult structures and in creating novel ideas for better use of proteins as drug targets. Experimental structures will still be needed to provide more detailed information about proteins and their interactions and dynamics as well as to support further developments in AI. Predictions and experiments will therefore complement each other and create an exciting time in the field of protein research to accelerate research and innovations.
Anastassios Papageorgiou
The writer is Head of Protein Structure and Chemistry core at Turku Bioscience Centre, Adjunct Professor at Faculty of Science, PhD